方法1 自帶
pytorch自帶方法,計(jì)算模型參數(shù)總量
total = sum([param.nelement() for param in model.parameters()])
print("Number of parameter: %.2fM" % (total/1e6))
或者
total = sum(p.numel() for p in model.parameters())
print("Total params: %.2fM" % (total/1e6))
方法2 編寫代碼
計(jì)算模型參數(shù)總量和模型計(jì)算量
def count_params(model, input_size=224):
# param_sum = 0
with open('models.txt', 'w') as fm:
fm.write(str(model))
# 計(jì)算模型的計(jì)算量
calc_flops(model, input_size)
# 計(jì)算模型的參數(shù)總量
model_parameters = filter(lambda p: p.requires_grad, model.parameters())
params = sum([np.prod(p.size()) for p in model_parameters])
print('The network has {} params.'.format(params))
# 計(jì)算模型的計(jì)算量
def calc_flops(model, input_size):
def conv_hook(self, input, output):
batch_size, input_channels, input_height, input_width = input[0].size()
output_channels, output_height, output_width = output[0].size()
kernel_ops = self.kernel_size[0] * self.kernel_size[1] * (self.in_channels / self.groups) * (
2 if multiply_adds else 1)
bias_ops = 1 if self.bias is not None else 0
params = output_channels * (kernel_ops + bias_ops)
flops = batch_size * params * output_height * output_width
list_conv.append(flops)
def linear_hook(self, input, output):
batch_size = input[0].size(0) if input[0].dim() == 2 else 1
weight_ops = self.weight.nelement() * (2 if multiply_adds else 1)
bias_ops = self.bias.nelement()
flops = batch_size * (weight_ops + bias_ops)
list_linear.append(flops)
def bn_hook(self, input, output):
list_bn.append(input[0].nelement())
def relu_hook(self, input, output):
list_relu.append(input[0].nelement())
def pooling_hook(self, input, output):
batch_size, input_channels, input_height, input_width = input[0].size()
output_channels, output_height, output_width = output[0].size()
kernel_ops = self.kernel_size * self.kernel_size
bias_ops = 0
params = output_channels * (kernel_ops + bias_ops)
flops = batch_size * params * output_height * output_width
list_pooling.append(flops)
def foo(net):
childrens = list(net.children())
if not childrens:
if isinstance(net, torch.nn.Conv2d):
net.register_forward_hook(conv_hook)
if isinstance(net, torch.nn.Linear):
net.register_forward_hook(linear_hook)
if isinstance(net, torch.nn.BatchNorm2d):
net.register_forward_hook(bn_hook)
if isinstance(net, torch.nn.ReLU):
net.register_forward_hook(relu_hook)
if isinstance(net, torch.nn.MaxPool2d) or isinstance(net, torch.nn.AvgPool2d):
net.register_forward_hook(pooling_hook)
return
for c in childrens:
foo(c)
multiply_adds = False
list_conv, list_bn, list_relu, list_linear, list_pooling = [], [], [], [], []
foo(model)
if '0.4.' in torch.__version__:
if assets.USE_GPU:
input = torch.cuda.FloatTensor(torch.rand(2, 3, input_size, input_size).cuda())
else:
input = torch.FloatTensor(torch.rand(2, 3, input_size, input_size))
else:
input = Variable(torch.rand(2, 3, input_size, input_size), requires_grad=True)
_ = model(input)
total_flops = (sum(list_conv) + sum(list_linear) + sum(list_bn) + sum(list_relu) + sum(list_pooling))
print(' + Number of FLOPs: %.2fM' % (total_flops / 1e6 / 2))
方法3 thop
需要安裝thop
調(diào)用方法:計(jì)算模型參數(shù)總量和模型計(jì)算量,而且會(huì)打印每一層網(wǎng)絡(luò)的具體信息
from thop import profile
input = torch.randn(1, 3, 224, 224)
flops, params = profile(model, inputs=(input,))
print(flops)
print(params)
或者
from torchvision.models import resnet50
from thop import profile
# model = resnet50()
checkpoints = '模型path'
model = torch.load(checkpoints)
model_name = 'yolov3 cut asff'
input = torch.randn(1, 3, 224, 224)
flops, params = profile(model, inputs=(input, ),verbose=True)
print("%s | %.2f | %.2f" % (model_name, params / (1000 ** 2), flops / (1000 ** 3)))#這里除以1000的平方,是為了化成M的單位,
注意:輸入必須是四維的
提高輸出可讀性, 加入一下代碼。
from thop import clever_format
macs, params = clever_format([flops, params], "%.3f")
方法4 torchstat
from torchstat import stat
from torchvision.models import resnet50, resnet101, resnet152, resnext101_32x8d
model = resnet50()
stat(model, (3, 224, 224)) # (3,224,224)表示輸入圖片的尺寸
使用torchstat這個(gè)庫(kù)來(lái)查看網(wǎng)絡(luò)模型的一些信息,包括總的參數(shù)量params、MAdd、顯卡內(nèi)存占用量和FLOPs等。需要安裝torchstat:
方法5 ptflops
作用:計(jì)算模型參數(shù)總量和模型計(jì)算量
安裝方法:pip install ptflops
或者
pip install --upgrade git+https://github.com/sovrasov/flops-counter.pytorch.git
使用方法
import torchvision.models as models
import torch
from ptflops import get_model_complexity_info
with torch.cuda.device(0):
net = models.resnet18()
flops, params = get_model_complexity_info(net, (3, 224, 224), as_strings=True, print_per_layer_stat=True) #不用寫batch_size大小,默認(rèn)batch_size=1
print('Flops: ' + flops)
print('Params: ' + params)
或者
from torchvision.models import resnet50
import torch
import torchvision.models as models
# import torch
from ptflops import get_model_complexity_info
# model = models.resnet50() #調(diào)用官方的模型,
checkpoints = '自己模型的path'
model = torch.load(checkpoints)
model_name = 'yolov3 cut'
flops, params = get_model_complexity_info(model, (3,320,320),as_strings=True,print_per_layer_stat=True)
print("%s |%s |%s" % (model_name,flops,params))
注意,這里輸入一定是要tuple類型,且不需要輸入batch,直接輸入輸入通道數(shù)量與尺寸,如(3,320,320) 320為網(wǎng)絡(luò)輸入尺寸。
輸出為網(wǎng)絡(luò)模型的總參數(shù)量(單位M,即百萬(wàn))與計(jì)算量(單位G,即十億)
方法6 torchsummary
安裝:pip install torchsummary
使用方法:
from torchsummary import summary
...
summary(your_model, input_size=(channels, H, W))
作用:
1、每一層的類型、shape 和 參數(shù)量
2、模型整體的參數(shù)量
3、模型大小,和 fp/bp 一次需要的內(nèi)存大小,可以用來(lái)估計(jì)最佳 batch_size
補(bǔ)充:pytorch計(jì)算模型算力與參數(shù)大小
ptflops介紹
官方鏈接
這個(gè)腳本設(shè)計(jì)用于計(jì)算卷積神經(jīng)網(wǎng)絡(luò)中乘法-加法操作的理論數(shù)量。它還可以計(jì)算參數(shù)的數(shù)量和打印給定網(wǎng)絡(luò)的每層計(jì)算成本。
支持layer:Conv1d/2d/3d,ConvTranspose2d,BatchNorm1d/2d/3d,激活(ReLU, PReLU, ELU, ReLU6, LeakyReLU),Linear,Upsample,Poolings (AvgPool1d/2d/3d、MaxPool1d/2d/3d、adaptive ones)
安裝要求:Pytorch >= 0.4.1, torchvision >= 0.2.1
get_model_complexity_info()
get_model_complexity_info是ptflops下的一個(gè)方法,可以計(jì)算出網(wǎng)絡(luò)的算力與模型參數(shù)大小,并且可以輸出每層的算力消耗。
栗子
以輸出Mobilenet_v2算力信息為例:
from ptflops import get_model_complexity_info
from torchvision import models
net = models.mobilenet_v2()
ops, params = get_model_complexity_info(net, (3, 224, 224), as_strings=True, print_per_layer_stat=True, verbose=True)
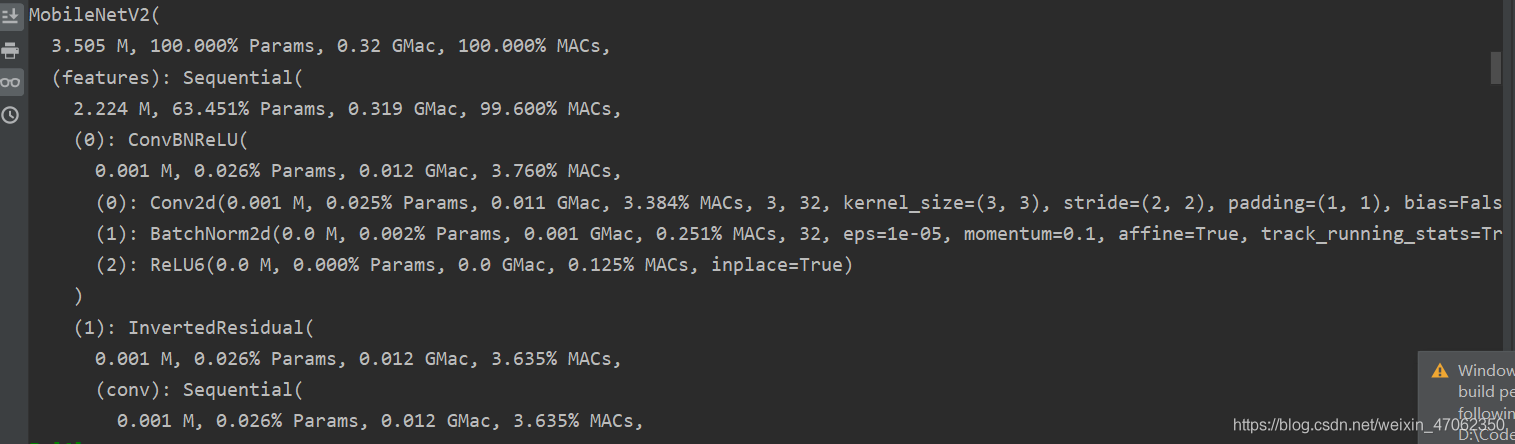
從圖中可以看到,MobileNetV2在輸入圖像尺寸為(3, 224, 224)的情況下將會(huì)產(chǎn)生3.505MB的參數(shù),算力消耗為0.32G,同時(shí)還打印出了每個(gè)層所占用的算力,權(quán)重參數(shù)數(shù)量。當(dāng)然,整個(gè)模型的算力大小與模型大小也被存到了變量ops與params中。
以上為個(gè)人經(jīng)驗(yàn),希望能給大家一個(gè)參考,也希望大家多多支持腳本之家。
您可能感興趣的文章:- PyTorch和Keras計(jì)算模型參數(shù)的例子
- pytorch 求網(wǎng)絡(luò)模型參數(shù)實(shí)例
- pytorch獲取模型某一層參數(shù)名及參數(shù)值方式