目錄
- 爬蟲Python驗(yàn)證碼識別
- 1、批量下載驗(yàn)證碼圖片
- 2、識別代碼看看效果
- 3、折騰降噪、去干擾
爬蟲Python驗(yàn)證碼識別
前言:
二值化、普通降噪、8鄰域降噪
tesseract、tesserocr、PIL
參考文獻(xiàn)--代碼地址:https://github.com/liguobao/python-verify-code-ocr
1、批量下載驗(yàn)證碼圖片
import shutil
import requests
from loguru import logger
for i in range(100):
url = 'http://xxxx/create/validate/image'
response = requests.get(url, stream=True)
with open(f'./imgs/{i}.png', 'wb') as out_file:
response.raw.decode_content = True
shutil.copyfileobj(response.raw, out_file)
logger.info(f"download {i}.png successfully.")
del response
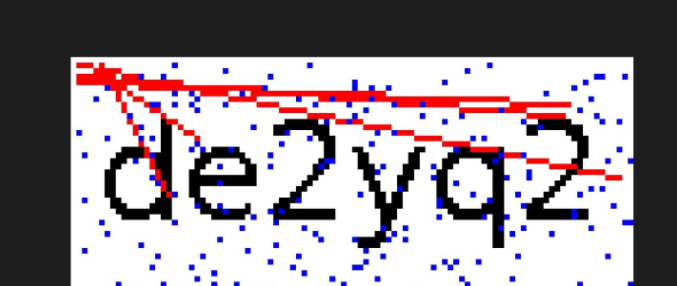
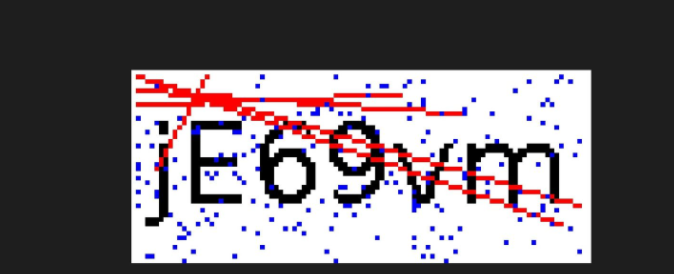
2、識別代碼看看效果
from PIL import Image
import tesserocr
img = Image.open("./imgs/98.png")
img.show()
img_l = img.convert("L")# 灰階圖
img_l.show()
verify_code1 = tesserocr.image_to_text(img)
verify_code2 = tesserocr.image_to_text(img_l)
print(f"verify_code1:{verify_code1}")
print(f"verify_code2:{verify_code2}")
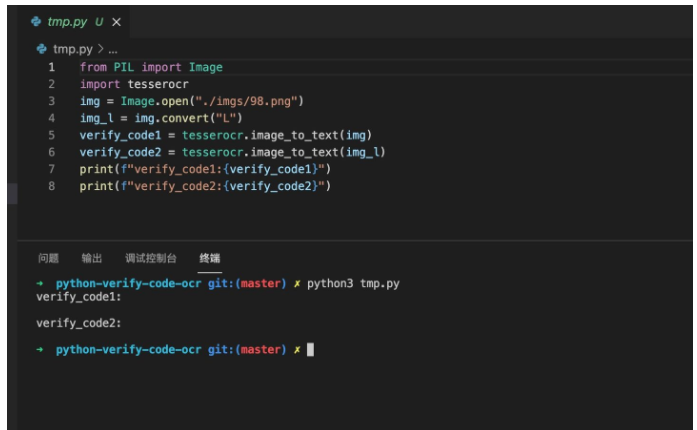
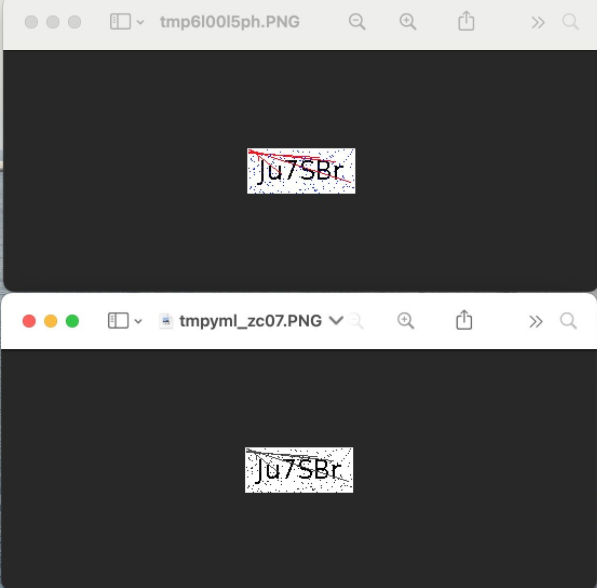
毫無疑問,無論是原圖還是灰階圖,一無所有。
3、折騰降噪、去干擾
Python圖片驗(yàn)證碼降噪 - 8鄰域降噪
from PIL import Image
# https://www.cnblogs.com/jhao/p/10345853.html Python圖片驗(yàn)證碼降噪 — 8鄰域降噪
def noise_remove_pil(image_name, k):
"""
8鄰域降噪
Args:
image_name: 圖片文件命名
k: 判斷閾值
Returns:
"""
def calculate_noise_count(img_obj, w, h):
"""
計(jì)算鄰域非白色的個數(shù)
Args:
img_obj: img obj
w: width
h: height
Returns:
count (int)
"""
count = 0
width, height = img_obj.size
for _w_ in [w - 1, w, w + 1]:
for _h_ in [h - 1, h, h + 1]:
if _w_ > width - 1:
continue
if _h_ > height - 1:
continue
if _w_ == w and _h_ == h:
continue
if img_obj.getpixel((_w_, _h_)) 230: # 這里因?yàn)槭腔叶葓D像,設(shè)置小于230為非白色
count += 1
return count
img = Image.open(image_name)
# 灰度
gray_img = img.convert('L')
w, h = gray_img.size
for _w in range(w):
for _h in range(h):
if _w == 0 or _h == 0:
gray_img.putpixel((_w, _h), 255)
continue
# 計(jì)算鄰域非白色的個數(shù)
pixel = gray_img.getpixel((_w, _h))
if pixel == 255:
continue
if calculate_noise_count(gray_img, _w, _h) k:
gray_img.putpixel((_w, _h), 255)
return gray_img
if __name__ == '__main__':
image = noise_remove_pil("./imgs/1.png", 4)
image.show()
看下圖效果:
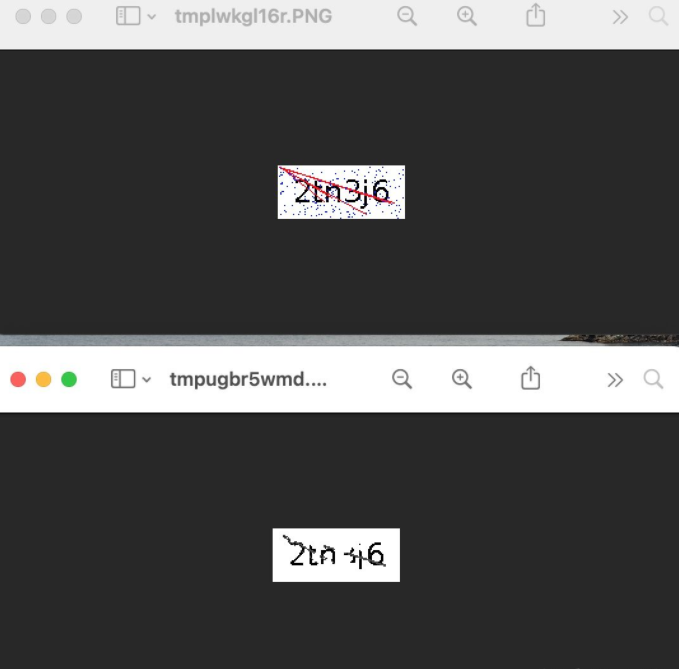
這樣差不多了,不過還可以提升
提升新思路:
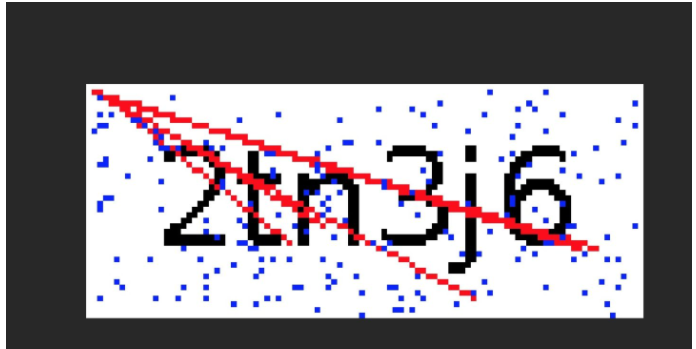
這邊的干擾線是從某個點(diǎn)發(fā)出來的紅色線條,
其實(shí)我只需要把紅色的像素點(diǎn)都干掉,這個線條也會被去掉。
from PIL import Image
import tesserocr
img = Image.open("./imgs/98.png")
img.show()
# 嘗試去掉紅像素點(diǎn)
w, h = img.size
for _w in range(w):
for _h in range(h):
o_pixel = img.getpixel((_w, _h))
if o_pixel == (255, 0, 0):
img.putpixel((_w, _h), (255, 255, 255))
img.show()
img_l = img.convert("L")
# img_l.show()
verify_code1 = tesserocr.image_to_text(img)
verify_code2 = tesserocr.image_to_text(img_l)
print(f"verify_code1:{verify_code1}")
print(f"verify_code2:{verify_code2}")
看起來OK,上面還有零星的藍(lán)色像素掉,也可以用同樣的方法一起去掉。
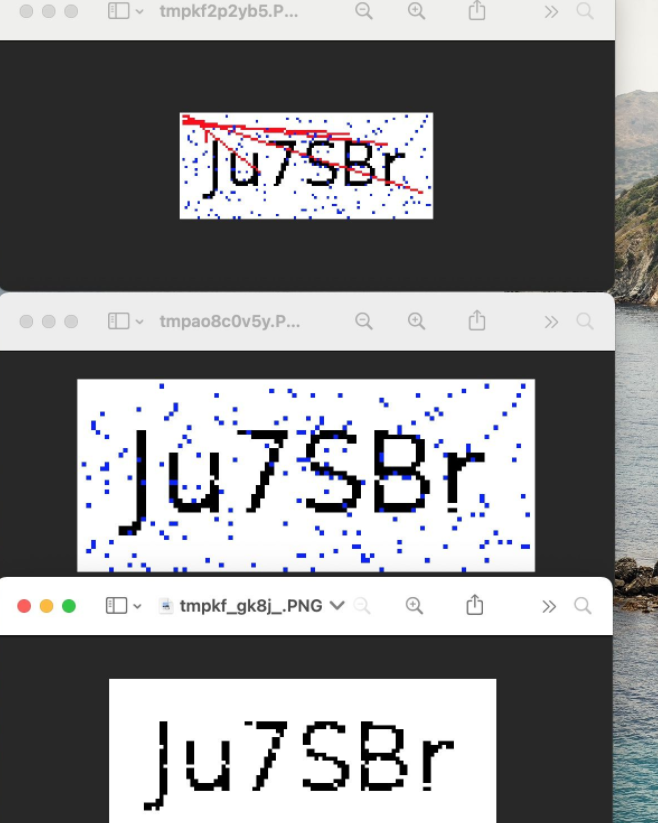
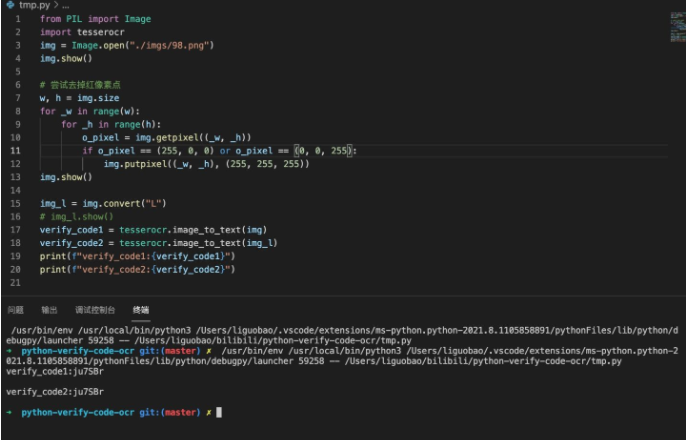
甚至OCR都直接出效果了
好了,完結(jié)撒花。
不過,后面發(fā)現(xiàn),有些紅色線段和藍(lán)色點(diǎn),是和驗(yàn)證碼重合的。
這個時候,如果直接填成白色,就容易把字母切開,導(dǎo)致識別效果變差。
當(dāng)前點(diǎn)是紅色或者藍(lán)色,判斷周圍點(diǎn)是不是超過兩個像素點(diǎn)是黑色。
是,填充為黑色。
否,填充成白色。
最終完整代碼:
from PIL import Image
import tesserocr
from loguru import logger
class VerfyCodeOCR():
def __init__(self) -> None:
pass
def ocr(self, img):
""" 驗(yàn)證碼OCR
Args:
img (img): imgObject/imgPath
Returns:
[string]: 識別結(jié)果
"""
img_obj = Image.open(img) if type(img) == str else img
self._remove_pil(img_obj)
verify_code = tesserocr.image_to_text(img_obj)
return verify_code.replace("\n", "").strip()
def _get_p_black_count(self, img: Image, _w: int, _h: int):
""" 獲取當(dāng)前位置周圍像素點(diǎn)中黑色元素的個數(shù)
Args:
img (img): 圖像信息
_w (int): w坐標(biāo)
_h (int): h坐標(biāo)
Returns:
int: 個數(shù)
"""
w, h = img.size
p_round_items = []
# 超過了橫縱坐標(biāo)
if _w == 0 or _w == w-1 or 0 == _h or _h == h-1:
return 0
p_round_items = [img.getpixel(
(_w, _h-1)), img.getpixel((_w, _h+1)), img.getpixel((_w-1, _h)), img.getpixel((_w+1, _h))]
p_black_count = 0
for p_item in p_round_items:
if p_item == (0, 0, 0):
p_black_count = p_black_count+1
return p_black_count
def _remove_pil(self, img: Image):
"""清理干擾識別的線條和噪點(diǎn)
Args:
img (img): 圖像對象
Returns:
[img]: 被清理過的圖像對象
"""
w, h = img.size
for _w in range(w):
for _h in range(h):
o_pixel = img.getpixel((_w, _h))
# 當(dāng)前像素點(diǎn)是紅色(線段) 或者 綠色(噪點(diǎn))
if o_pixel == (255, 0, 0) or o_pixel == (0, 0, 255):
# 周圍黑色數(shù)量大于2,則把當(dāng)前像素點(diǎn)填成黑色;否則用白色覆蓋
p_black_count = self._get_p_black_count(img, _w, _h)
if p_black_count >= 2:
img.putpixel((_w, _h), (0, 0, 0))
else:
img.putpixel((_w, _h), (255, 255, 255))
logger.info(f"_remove_pil finish.")
# img.show()
return img
if __name__ == '__main__':
verfyCodeOCR = VerfyCodeOCR()
img_path = "./imgs/51.png"
img= Image.open(img_path)
img.show()
ocr_result = verfyCodeOCR.ocr(img)
img.show()
logger.info(ocr_result)
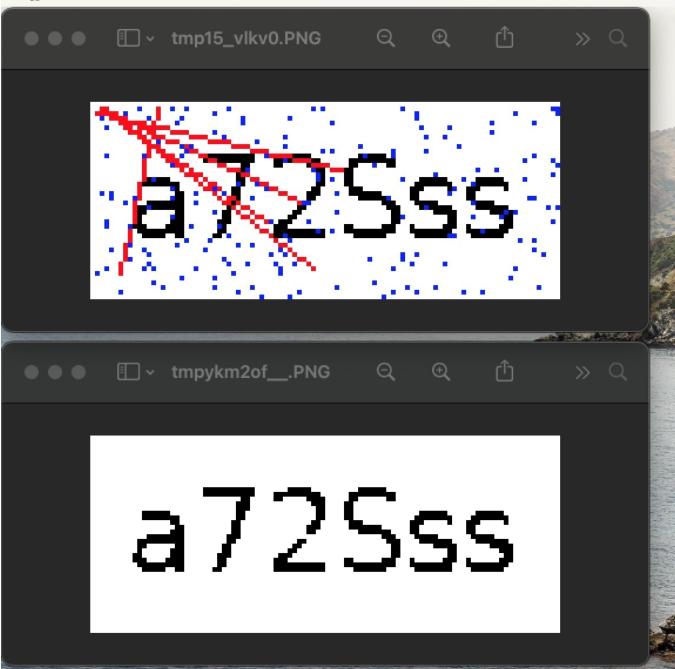
到此這篇關(guān)于爬蟲Python驗(yàn)證碼識別入門的文章就介紹到這了,更多相關(guān)Python驗(yàn)證碼識別內(nèi)容請搜索腳本之家以前的文章或繼續(xù)瀏覽下面的相關(guān)文章希望大家以后多多支持腳本之家!
您可能感興趣的文章:- 關(guān)于python爬蟲應(yīng)用urllib庫作用分析
- python爬蟲Scrapy框架:媒體管道原理學(xué)習(xí)分析
- python爬蟲Mitmproxy安裝使用學(xué)習(xí)筆記
- Python爬蟲和反爬技術(shù)過程詳解
- python爬蟲之Appium爬取手機(jī)App數(shù)據(jù)及模擬用戶手勢
- Python爬蟲技術(shù)
- Python爬蟲爬取商品失敗處理方法
- Python獲取江蘇疫情實(shí)時數(shù)據(jù)及爬蟲分析
- Python爬蟲之Scrapy環(huán)境搭建案例教程
- Python爬蟲中urllib3與urllib的區(qū)別是什么
- 教你如何利用python3爬蟲爬取漫畫島-非人哉漫畫
- Python爬蟲分析匯總