threshold:固定閾值二值化,
ret, dst = cv2.threshold(src, thresh, maxval, type)
- src: 輸入圖,只能輸入單通道圖像,通常來說為灰度圖
- dst: 輸出圖
- thresh: 閾值
- maxval: 當(dāng)像素值超過了閾值(或者小于閾值,根據(jù)type來決定),所賦予的值
- type:二值化操作的類型,包含以下5種類型: cv2.THRESH_BINARY; cv2.THRESH_BINARY_INV; cv2.THRESH_TRUNC; cv2.THRESH_TOZERO;cv2.THRESH_TOZERO_INV
官方文檔的示例代碼:
import cv2
import numpy as np
from matplotlib import pyplot as plt
img = cv2.imread('gradient.png',0)
ret,thresh1 = cv2.threshold(img,127,255,cv2.THRESH_BINARY)
ret,thresh2 = cv2.threshold(img,127,255,cv2.THRESH_BINARY_INV)
ret,thresh3 = cv2.threshold(img,127,255,cv2.THRESH_TRUNC)
ret,thresh4 = cv2.threshold(img,127,255,cv2.THRESH_TOZERO)
ret,thresh5 = cv2.threshold(img,127,255,cv2.THRESH_TOZERO_INV)
titles = ['Original Image','BINARY','BINARY_INV','TRUNC','TOZERO','TOZERO_INV']
images = [img, thresh1, thresh2, thresh3, thresh4, thresh5]
for i in xrange(6):
plt.subplot(2,3,i+1),plt.imshow(images[i],'gray')
plt.title(titles[i])
plt.xticks([]),plt.yticks([])
plt.show()
結(jié)果為:
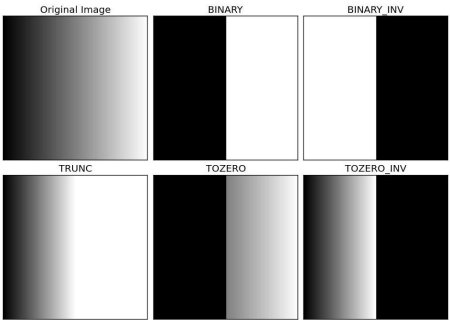
adaptiveThreshold:自適應(yīng)閾值二值化
自適應(yīng)閾值二值化函數(shù)根據(jù)圖片一小塊區(qū)域的值來計(jì)算對應(yīng)區(qū)域的閾值,從而得到也許更為合適的圖片。
dst = cv2.adaptiveThreshold(src, maxval, thresh_type, type, Block Size, C)
- src: 輸入圖,只能輸入單通道圖像,通常來說為灰度圖
- dst: 輸出圖
- maxval: 當(dāng)像素值超過了閾值(或者小于閾值,根據(jù)type來決定),所賦予的值
- thresh_type: 閾值的計(jì)算方法,包含以下2種類型:cv2.ADAPTIVE_THRESH_MEAN_C; cv2.ADAPTIVE_THRESH_GAUSSIAN_C.
- type:二值化操作的類型,與固定閾值函數(shù)相同,包含以下5種類型: cv2.THRESH_BINARY; cv2.THRESH_BINARY_INV; cv2.THRESH_TRUNC; cv2.THRESH_TOZERO;cv2.THRESH_TOZERO_INV.
- Block Size: 圖片中分塊的大小
- C :閾值計(jì)算方法中的常數(shù)項(xiàng)
官方文檔的示例代碼:
import cv2
import numpy as np
from matplotlib import pyplot as plt
img = cv2.imread('sudoku.png',0)
img = cv2.medianBlur(img,5)
ret,th1 = cv2.threshold(img,127,255,cv2.THRESH_BINARY)
th2 = cv2.adaptiveThreshold(img,255,cv2.ADAPTIVE_THRESH_MEAN_C,\
cv2.THRESH_BINARY,11,2)
th3 = cv2.adaptiveThreshold(img,255,cv2.ADAPTIVE_THRESH_GAUSSIAN_C,\
cv2.THRESH_BINARY,11,2)
titles = ['Original Image', 'Global Thresholding (v = 127)',
'Adaptive Mean Thresholding', 'Adaptive Gaussian Thresholding']
images = [img, th1, th2, th3]
for i in xrange(4):
plt.subplot(2,2,i+1),plt.imshow(images[i],'gray')
plt.title(titles[i])
plt.xticks([]),plt.yticks([])
plt.show()
結(jié)果為:
Otsu's Binarization: 基于直方圖的二值化
Otsu's Binarization是一種基于直方圖的二值化方法,它需要和threshold函數(shù)配合使用。
Otsu過程:
1. 計(jì)算圖像直方圖;
2. 設(shè)定一閾值,把直方圖強(qiáng)度大于閾值的像素分成一組,把小于閾值的像素分成另外一組;
3. 分別計(jì)算兩組內(nèi)的偏移數(shù),并把偏移數(shù)相加;
4. 把0~255依照順序多為閾值,重復(fù)1-3的步驟,直到得到最小偏移數(shù),其所對應(yīng)的值即為結(jié)果閾值。
官方文檔的示例代碼:
import cv2
import numpy as np
from matplotlib import pyplot as plt
img = cv2.imread('noisy2.png',0)
# global thresholding
ret1,th1 = cv2.threshold(img,127,255,cv2.THRESH_BINARY)
# Otsu's thresholding
ret2,th2 = cv2.threshold(img,0,255,cv2.THRESH_BINARY+cv2.THRESH_OTSU)
# Otsu's thresholding after Gaussian filtering
blur = cv2.GaussianBlur(img,(5,5),0)
ret3,th3 = cv2.threshold(blur,0,255,cv2.THRESH_BINARY+cv2.THRESH_OTSU)
# plot all the images and their histograms
images = [img, 0, th1,
img, 0, th2,
blur, 0, th3]
titles = ['Original Noisy Image','Histogram','Global Thresholding (v=127)',
'Original Noisy Image','Histogram',"Otsu's Thresholding",
'Gaussian filtered Image','Histogram',"Otsu's Thresholding"]
for i in xrange(3):
plt.subplot(3,3,i*3+1),plt.imshow(images[i*3],'gray')
plt.title(titles[i*3]), plt.xticks([]), plt.yticks([])
plt.subplot(3,3,i*3+2),plt.hist(images[i*3].ravel(),256)
plt.title(titles[i*3+1]), plt.xticks([]), plt.yticks([])
plt.subplot(3,3,i*3+3),plt.imshow(images[i*3+2],'gray')
plt.title(titles[i*3+2]), plt.xticks([]), plt.yticks([])
plt.show()
結(jié)果為:
參考文獻(xiàn):http://docs.opencv.org/3.2.0/d7/d4d/tutorial_py_thresholding.html
到此這篇關(guān)于opencv函數(shù)threshold、adaptiveThreshold、Otsu二值化的實(shí)現(xiàn)的文章就介紹到這了,更多相關(guān)opencv threshold、adaptiveThreshold、Otsu內(nèi)容請搜索腳本之家以前的文章或繼續(xù)瀏覽下面的相關(guān)文章希望大家以后多多支持腳本之家!
您可能感興趣的文章:- OpenCV 使用imread()函數(shù)讀取圖片的六種正確姿勢
- python+opencv邊緣提取與各函數(shù)參數(shù)解析
- 詳解opencv中畫圓circle函數(shù)和橢圓ellipse函數(shù)
- 使用OpenCV circle函數(shù)圖像上畫圓的示例代碼
- Python OpenCV 使用滑動條來調(diào)整函數(shù)參數(shù)的方法
- Opencv2.4.9函數(shù)HoughLinesP分析
- OpenCV中的cv::Mat函數(shù)將數(shù)據(jù)寫入txt文件